In this activity, we would be using 2 different algorithms for automatic white balancing. Reference white balancing is done by picking in the image the color that would represent white (reference white). The RGB values of this white would then be used as a divisor or balancing constants for the respective channels. Gray world algorithm is done by taking the average of each channel and then using these averages as the balancing constants.
These 2 algorithms were performed on images taken at different white balance of the camera. The white balance camera settings used were fluorescentH (which is redder compared to the bluer fluorescent setting), daylight, and tungsten.
FluorescentH
Daylight
Tungsten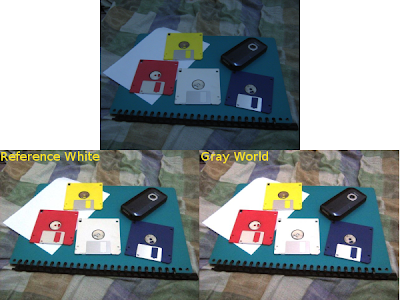
Both algorithms seems to perform relatively well, although the gray world algorithm needs some more tweaking in order to lower the brightness.
After trying both algorithms on colorful images, we then tried both on an image that contains few colors.
Blue Objects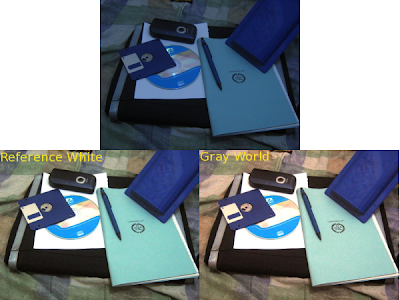
For low number of colors, the reference white algorithm seems to perform better. The gray world algorithm produced an image which is more reddish than the image produced by the reference white algorithm. I think reference white algorithm is better for images with few colors since it uses a fixed white color as the balancing factor unlike the gray world which averages all the colors present which would not produce a good result since it render colors biased to some colors.
//Scilab CodeI = imread(filename + ".JPG");//Reference Whitemethod = "rw-";imshow(I);pix = locate(1);Rw = I(pix(1), pix(2), 1);Gw = I(pix(1), pix(2), 2);Bw = I(pix(1), pix(2), 3);clf();//Gray World//method = "gw-";//Rw = mean(I(:, :, 1));//Gw = mean(I(:, :, 2));//Bw = mean(I(:, :, 3));I(:, :, 1) = I(:, :, 1)/Rw;I(:, :, 2) = I(:, :, 2)/Gw;I(:, :, 3) = I(:, :, 3)/Bw;//I = I * 0.5; // for Gray world algorithm to reduce saturationI(I > 1.0) = 1.0;//code endFor this activity, I give myself a grade of 10 since all the objectives were accomplished. :)
Collaborators: Raf Jaculbia